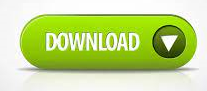
Raw accelerometry data were collected from a thigh-worn accelerometer during a 20-min simulated drive (8:10 h and 17:30 h each day). Participants (n = 84, Mean ± SD age = 23.5 ± 4.8, 49% Female) completed a seven-day laboratory study. This study aimed to apply deep learning to raw accelerometry data collected during a simulated driving task to classify recent sitting and sleep history. Therefore, objective knowledge of a driver’s recent sitting and sleep history could help reduce safety risks. Prolonged sitting and inadequate sleep can impact driving performance. Moreover, the latent fatigue features extracted by deep learning methods have been demonstrated to be effective for fatigue detection. We conducted experiments to show that the fatigue features extracted by Convolutional Neural Networks are superior to traditional handcrafted ones while single features cannot guarantee robustness. Finally, we present the work on integration of RGB-D camera and deep learning, where Generative Adversarial Networks and multi-channel schemes are utilized to enhance the performance. Then, we focus on RGB-D camera and deep learning which are two state-of-the-art solutions in this field.
#Driverdr display driver uninstaller review driver#
Firstly, we analyze and discuss four types of different fatigue detection technologies based on driver physiological signals, behavior features, vehicle running features, and information fusion, respectively. In this review, we summarize the latest research findings and analyze the developmental trends of driver fatigue detection. Our direct driver sleepiness detection system developed from the integration of biophysiological information and internal validation provides a valuable algorithm, specifically toward alertness level.ĭriver fatigue is an essential reason for traffic accidents, which poses a severe threat to people's lives and property. The ANN with DFT combined with the EAR, MAR, and FL offered the best performance.
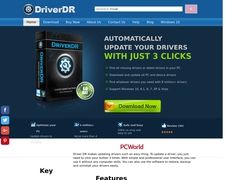
In addition, by applying the discrete Fourier transform (DFT) to the composite features, the ANN combined with the EAR and MAR features again had the highest sensitivity (72.25%), while the ANN combined with the EAR, MAR, and FL features had the highest accuracy and specificity (60.40% and 54.10%, respectively). The ANN combined with the EAR and MAR features had the most sensitivity (70.12%) while the ANN combined with the EAR, MAR, and FL features had the most accuracy and specificity (60.76% and 58.71%, respectively). Composite features of facial descriptors (ie, eye aspect ratio (EAR), mouth aspect ratio (MAR), face length (FL), and face width balance (FWB)) extracted from two-second video frames were investigated.
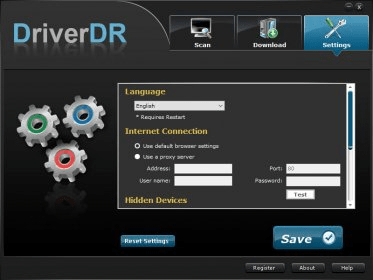
Artificial neural networks (ANN) were adopted as the model. The data were obtained from 10 participants. In this study, we developed an early drowsiness detection algorithm and device based on the "gold standard brain biophysiological signal" and facial expression digital data. Recent technologies for detection and alarm within automobiles for this condition are limited by their reliability, practicality, cost, and lack of clinical validation. A number of road accidents might then be avoided if an alert is sent to a driver that is deemed drowsy.ĭriving while drowsy is a major cause of traffic accidents globally. We conclude that by designing a hybrid drowsiness detection system that combines non-intusive physiological measures with other measures one would accurately determine the drowsiness level of a driver. The various ways through which drowsiness has been experimentally manipulated is also discussed. In this paper, we review these three measures as to the sensors used and discuss the advantages and limitations of each. A detailed review on these measures will provide insight on the present systems, issues associated with them and the enhancements that need to be done to make a robust system. Researchers have attempted to determine driver drowsiness using the following measures: (1) vehicle-based measures (2) behavioral measures and (3) physiological measures. Statistics indicate the need of a reliable driver drowsiness detection system which could alert the driver before a mishap happens. In recent years, driver drowsiness has been one of the major causes of road accidents and can lead to severe physical injuries, deaths and significant economic losses.
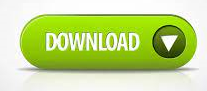